AUEB STATS Christmas SEMINAR 22/12/2017: Scalable Bayesian regression in high dimensions with multiple data sources by Konstantinos Perrakis (DZNE, Bonn)
Mon 4 Dec 2017 - 14:16
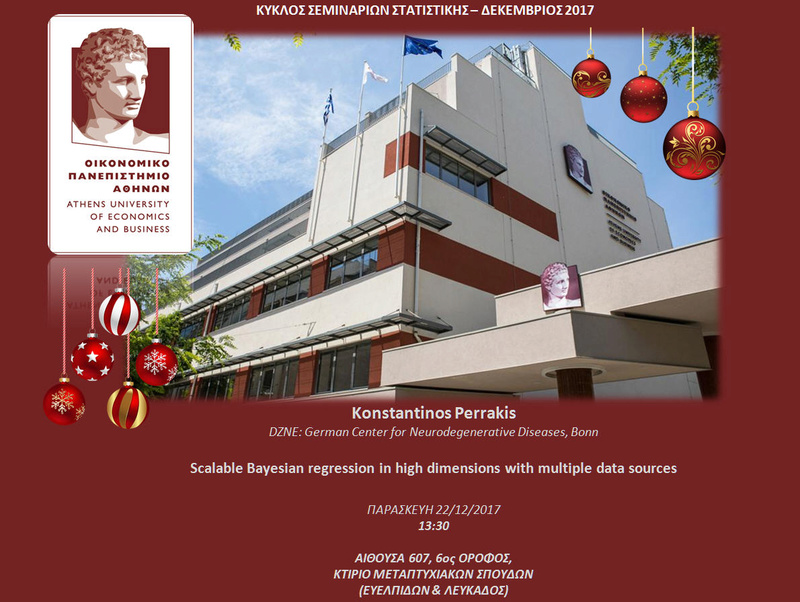
ΚΥΚΛΟΣ ΣΕΜΙΝΑΡΙΩΝ ΣΤΑΤΙΣΤΙΚΗΣ ΔΕΚΕΜΒΡΙΟΣ 2017
Konstantinos Perrakis
DZNE: German Center for Neurodegenerative Diseases, Bonn
Scalable Bayesian regression in high dimensions with multiple data sources
ΠΑΡΑΣΚΕΥΗ 22/12/2017
13:30
ΑΙΘΟΥΣΑ 607, 6ος ΟΡΟΦΟΣ,
ΚΤΙΡΙΟ ΜΕΤΑΠΤΥΧΙΑΚΩΝ ΣΠΟΥΔΩΝ
(ΕΥΕΛΠΙΔΩΝ & ΛΕΥΚΑΔΟΣ)
ΠΕΡΙΛΗΨΗ
Many current applications of high-dimensional regression involve multiple sources of covariates. We propose methodology for this setting, motivated by biomedical applications in the "wide data" regime with very large total dimensionality p and sample size n << p. As a starting point, we formulate a flexible ridge-type prior with shrinkage levels that are specific to data type or source. These multiple shrinkage levels are set automatically in a data-driven manner using empirical Bayes. Importantly, all the proposed estimators can be formulated in terms of outer-product data matrices of size n x n, rendering computation fast and scalable in the wide data setting, and are free of user-set tuning parameters. We extend the approaches towards sparse solutions via constrained minimization of a certain Kullback-Leibler divergence, including a relaxed variant that scales to large p, allows adaptive and source-specific shrinkage and has a closed-form solution. The proposed methods are compared to standard high-dimensional methods in a simulation study based on biological data. We present also results from a case study in Alzheimer's disease involving millions of predictors and multiple data sources.
- AUEB STATS SEMINARS 20/12/2019: Latent group structure and regularized regression by Konstantinos Perrakis
- AUEB STATS SEMINARS 8/6/2017: Scalable Approximation Algorithms for Bayesian Variable Selection by Feng Liang
- AUEB STATS SEMINARS 23/11/2017: High-dimensional model building for Spatial Regression by Taps Maiti (Michigan State University)
- AUEB SEMINARS - 28/4/2015: Integration of data from multiple sources: meta-analysis and synthesis analysis
- AUEB Stats Seminars 25/2/2022: Subset selection for big data regression: an improved approach
Permissions in this forum:
You cannot reply to topics in this forum