AUEB STATS SEMINARS 8/11/2018: Bayesian model selection for exponential random graph models via adjusted pseudolikelihoods by Lampros Bouranis
Tue 30 Oct 2018 - 17:53
AUEB STATISTICS SEMINAR SERIES NOVEMBER 2018
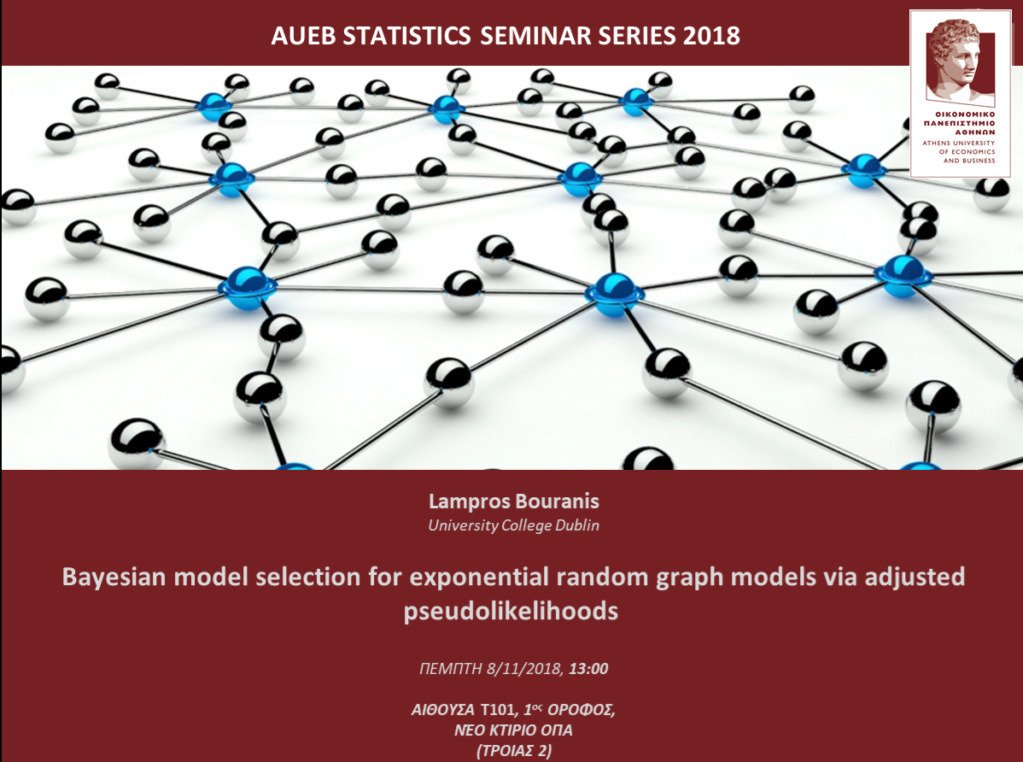
Lampros Bouranis, PhD
University College Dublin
Bayesian model selection for exponential random graph models via adjusted pseudolikelihoods
THURSDAY 8/11/2018
13:00
ROOM T101, 1ST FLOOR,
NEW AUEB BUILDING, (TROIAS 2)
ABSTRACT
Models with intractable likelihood functions arise in areas including network analysis and spatial statistics, especially those involving Gibbs random fields. Posterior parameter estimation in these settings is termed a doubly intractable problem because both the likelihood function and the posterior distribution are intractable. The comparison of Bayesian models is often based on the statistical evidence, the integral of the un-normalized posterior distribution over the model parameters which is rarely available in closed form. For doubly intractable models, estimating the evidence adds another layer of difficulty. Consequently, the selection of the model that best describes an observed network among a collection of exponential random graph models for network analysis is a daunting task. Pseudolikelihoods offer a tractable approximation to the likelihood but should be treated with caution because they can lead to an unreasonable inference. This talk specifies a method to adjust pseudolikelihoods to obtain a reasonable, yet tractable, approximation to the likelihood. This allows implementation of widely used computational methods for evidence estimation and pursuit of Bayesian model selection of exponential random graph models for the analysis of social networks. Empirical comparisons to existing methods show that our procedure yields similar evidence estimates, but at a lower computational cost.
FB event: https://www.facebook.com/events/498325764003335/
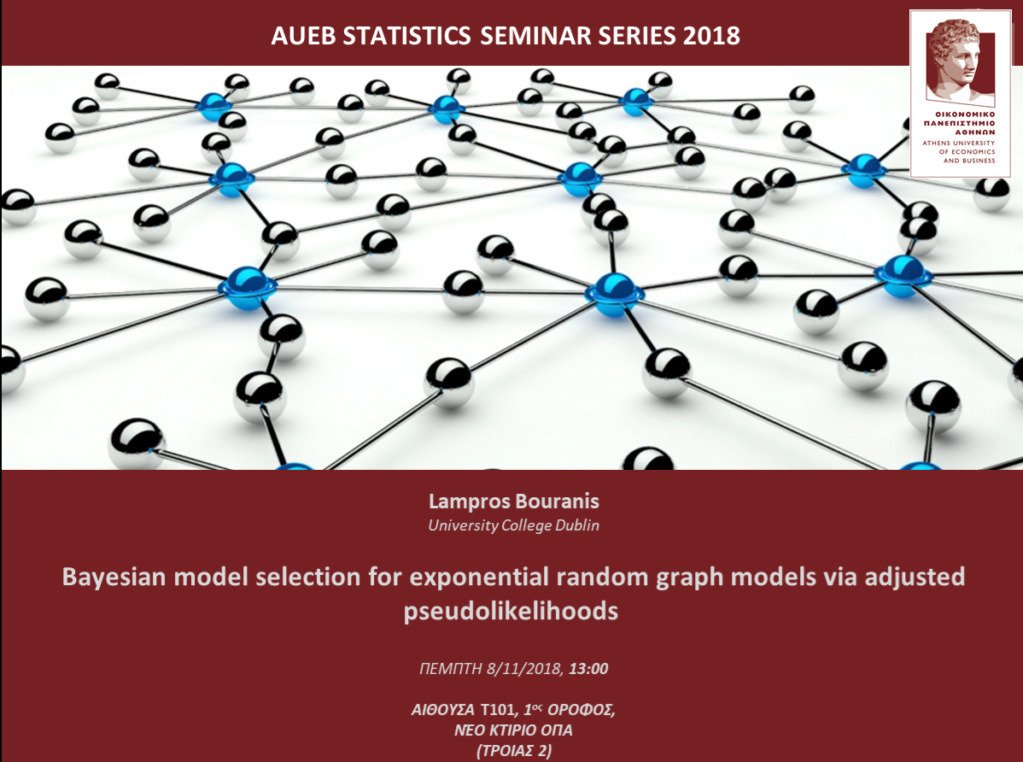
Lampros Bouranis, PhD
University College Dublin
Bayesian model selection for exponential random graph models via adjusted pseudolikelihoods
THURSDAY 8/11/2018
13:00
ROOM T101, 1ST FLOOR,
NEW AUEB BUILDING, (TROIAS 2)
ABSTRACT
Models with intractable likelihood functions arise in areas including network analysis and spatial statistics, especially those involving Gibbs random fields. Posterior parameter estimation in these settings is termed a doubly intractable problem because both the likelihood function and the posterior distribution are intractable. The comparison of Bayesian models is often based on the statistical evidence, the integral of the un-normalized posterior distribution over the model parameters which is rarely available in closed form. For doubly intractable models, estimating the evidence adds another layer of difficulty. Consequently, the selection of the model that best describes an observed network among a collection of exponential random graph models for network analysis is a daunting task. Pseudolikelihoods offer a tractable approximation to the likelihood but should be treated with caution because they can lead to an unreasonable inference. This talk specifies a method to adjust pseudolikelihoods to obtain a reasonable, yet tractable, approximation to the likelihood. This allows implementation of widely used computational methods for evidence estimation and pursuit of Bayesian model selection of exponential random graph models for the analysis of social networks. Empirical comparisons to existing methods show that our procedure yields similar evidence estimates, but at a lower computational cost.
FB event: https://www.facebook.com/events/498325764003335/
- AUEB STATS SEMINARS 20/2/2020: Bayesian Variable Selection for the Ising Network Model with Applications in Psychology by Maarten Marsman
- AUEB Stats Seminars 15/10/2021: Statistical Network Analysis with Bergm by Lampros Bouranis (AUEB)
- AUEB STATS SEMINARS 29/11/2018: Pivotal methods for Bayesian mixture models and classical k-means algorithm initialization by Leonardo Egidi
- AUEB STATS SEMINARS 18/9/2019: Bayesian analysis of multifidelity computer models with local features and non-nested experimental designs: Application to the WRF model by Georgios Karagiannis
- AUEB Stats Seminars 8/10/2021: From here to infinity - bridging finite and Bayesian nonparametric mixture models in model-based clustering by Sylvia Frühwirth-Schnatter (WU Vienna University of Economics and Busin
Permissions in this forum:
You cannot reply to topics in this forum