AUEB Stats Seminars 2/11/2022: Non-linear Network Autoregression by K. Fokianos (University of Cyprus)
Tue 1 Nov 2022 - 12:00
AUEB STATISTICS SEMINAR SERIES NOVEMBER 2022
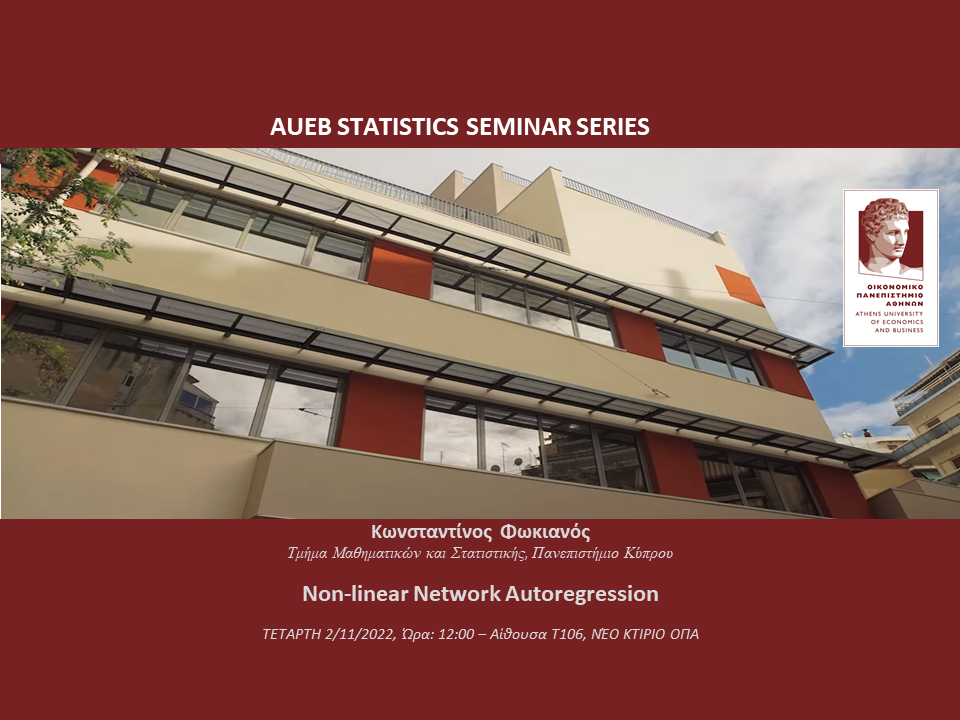
Konstantinos Fokianos
Department of Mathematics and Statistics, Cyprus University
Non-linear Network Autoregression
WEDNESDAY 2/11/2022
12:00
ROOM T106, NEW AUEB BUILDING
ABSTRACT
We study general nonlinear models for time series networks of integer and continuous valued data. The vector of high dimensional responses, measured on the nodes of a known network, is regressed non-linearly on its lagged value and on lagged values of the connected nodes by employing an appropriate smooth link function. We study stability conditions for such multivariate process and develop quasi maximum likelihood inference when the network dimension is increasing. In addition, under the same setup, we study linearity score tests by treating separately the cases of identifiable and non-identifiable parameters. In the case of identifiability, the test statistic converges to a chisquare distribution. However, when the parameters are not-identifiable, we develop a sup-type test whose p-values are approximated using a feasible bound and bootstrap methodology. Simulations and data examples complement the presentation.
This is a joint work with M. Armillotta.
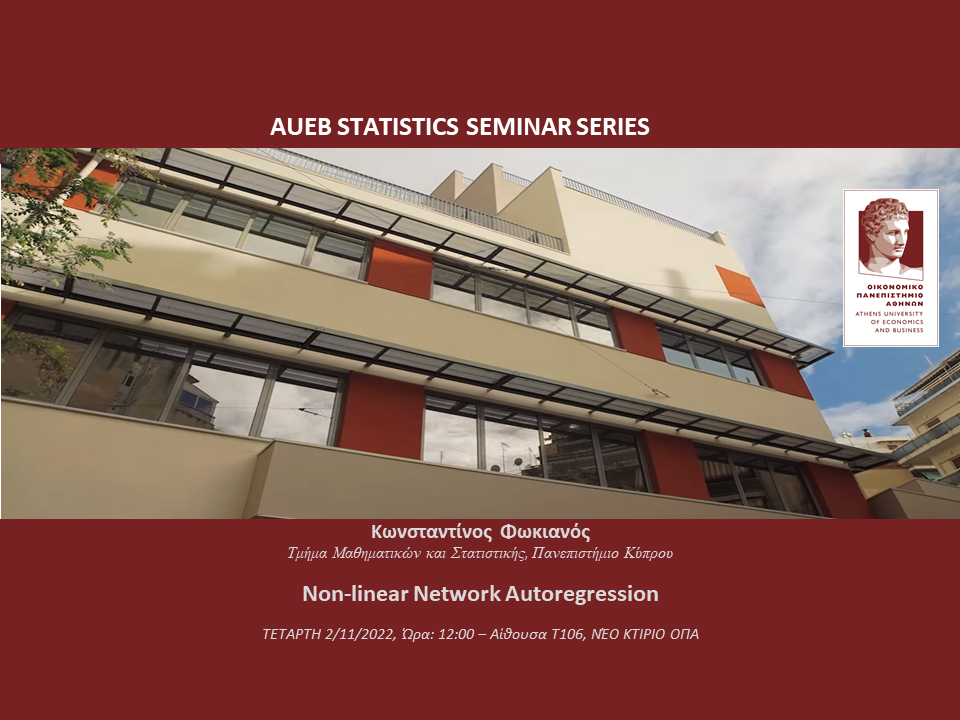
Konstantinos Fokianos
Department of Mathematics and Statistics, Cyprus University
Non-linear Network Autoregression
WEDNESDAY 2/11/2022
12:00
ROOM T106, NEW AUEB BUILDING
ABSTRACT
We study general nonlinear models for time series networks of integer and continuous valued data. The vector of high dimensional responses, measured on the nodes of a known network, is regressed non-linearly on its lagged value and on lagged values of the connected nodes by employing an appropriate smooth link function. We study stability conditions for such multivariate process and develop quasi maximum likelihood inference when the network dimension is increasing. In addition, under the same setup, we study linearity score tests by treating separately the cases of identifiable and non-identifiable parameters. In the case of identifiability, the test statistic converges to a chisquare distribution. However, when the parameters are not-identifiable, we develop a sup-type test whose p-values are approximated using a feasible bound and bootstrap methodology. Simulations and data examples complement the presentation.
This is a joint work with M. Armillotta.
- AUEB Stats Seminars 8/4/2022: A Bayesian Metafrontier Stochastic Model: A cross-country comparison by Sonia Malefaki (University of Patras)
- AUEB Stats Seminars 27/6/2022: Statistical Foundation of Deep Learning: Application to Big Data by Taps Maiti (Michigan State University)
- AUEB Stats Seminars 18/3/2022: Hypothesis Testing for the Covariance Matrix in High-Dimensional Transposable Data with Kronecker Product Dependence Structure by Anestis Touloumis (University of Brighton)
- AUEB Stats Seminars 11/2/2022: "Assessing competitive balance in the English First Division/Premier League for over forty seasons using a stochastic block model" by Nial Friel (University College Dublin)
- AUEB Stats Seminars 15/10/2021: Statistical Network Analysis with Bergm by Lampros Bouranis (AUEB)
Permissions in this forum:
You cannot reply to topics in this forum
|
|