AUEB STATS SEMINARS 23/11/2017: High-dimensional model building for Spatial Regression by Taps Maiti (Michigan State University)
Mon 23 Oct 2017 - 23:21
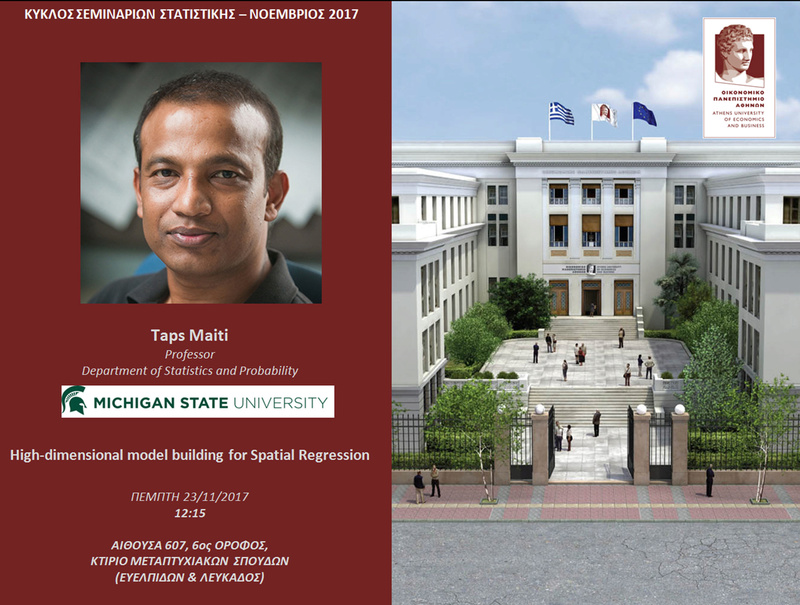
ΚΥΚΛΟΣ ΣΕΜΙΝΑΡΙΩΝ ΣΤΑΤΙΣΤΙΚΗΣ ΝΟΕΜΒΡΙΟΣ 2017
Taps Maiti
Professor, Graduate Director, Department of Statistics and Probability, Michigan State University
High-dimensional model building for Spatial Regression
ΠΕΜΠΤΗ 23/11/2017
12:15
ΑΙΘΟΥΣΑ 607, 6ος ΟΡΟΦΟΣ,
ΚΤΙΡΙΟ ΜΕΤΑΠΤΥΧΙΑΚΩΝ ΣΠΟΥΔΩΝ
(ΕΥΕΛΠΙΔΩΝ & ΛΕΥΚΑΔΟΣ)
ΠΕΡΙΛΗΨΗ
Spatial regression is an important predictive tool in many scientific applications and an additive model provides a flexible regression relationship between predictors and a response variable. Such model is proved to be effective in regression based prediction. In this talk, we develop a regularized variable selection technique for building a spatial additive model. We found that the approaches developed for independent data do not work well for spatially dependent data. This motivates us to propose a spatially weighted L-2 error norm with a group LASSO type penalty to select additive components for spatial additive models. We establish the selection consistency of the proposed approach where the penalty parameter depends on several factors, such as the order of approximation of additive components, characteristics of the spatial weight and spatial dependence, etc. An extensive simulation study provides a vivid picture of the impacts of dependent data structures, the choice of a spatial weight as well as the asymptotic behavior of the estimates. We also investigate other regression models in the context of spatially dependent data.
AUEB STATISTICS SEMINAR SERIES NOVEMBER 2017
Taps Maiti
Professor, Graduate Director, Department of Statistics and Probability, Michigan State University
High-dimensional model building for Spatial Regression
Thursday 23/11/2017
12:15
ROOM 607, 6th FLOOR,
POSTGRADUATE STUDIES BUILDING
(EVELPIDON & LEFKADOS)
ABSTRACT
Spatial regression is an important predictive tool in many scientific applications and an additive model provides a flexible regression relationship between predictors and a response variable. Such model is proved to be effective in regression based prediction. In this talk, we develop a regularized variable selection technique for building a spatial additive model. We found that the approaches developed for independent data do not work well for spatially dependent data. This motivates us to propose a spatially weighted L-2 error norm with a group LASSO type penalty to select additive components for spatial additive models. We establish the selection consistency of the proposed approach where the penalty parameter depends on several factors, such as the order of approximation of additive components, characteristics of the spatial weight and spatial dependence, etc. An extensive simulation study provides a vivid picture of the impacts of dependent data structures, the choice of a spatial weight as well as the asymptotic behavior of the estimates. We also investigate other regression models in the context of spatially dependent data.
- AUEB Stats Seminars 27/6/2022: Statistical Foundation of Deep Learning: Application to Big Data by Taps Maiti (Michigan State University)
- AUEB Stats Seminars 18/3/2022: Hypothesis Testing for the Covariance Matrix in High-Dimensional Transposable Data with Kronecker Product Dependence Structure by Anestis Touloumis (University of Brighton)
- AUEB SEMINARS - 1/4/2015: Integrating high-dimensional estimation and model selection with non-local priors
- AUEB Stats Seminars 24/6/2021: Nonparametric and high-dimensional functional graphical models by E. Solea
- AUEB STATS SEMINARS 21/2/2019: High-Dimensional Macroeconomic Forecasting Using Message Passing Algorithms by Dimitris Korobilis
Permissions in this forum:
You cannot reply to topics in this forum
|
|