AUEB STATS SEMINARS 30/3/2018: Estimating metabolite networks using a multi-step network approach that integrates information from lower leveled omic graphical structures by Georgios Bartzis
Tue 13 Mar 2018 - 11:28
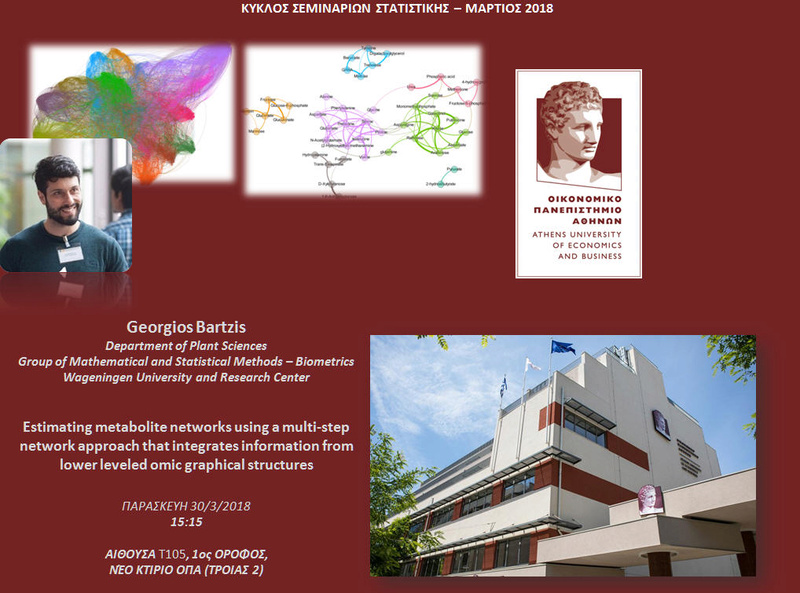
ΚΥΚΛΟΣ ΣΕΜΙΝΑΡΙΩΝ ΣΤΑΤΙΣΤΙΚΗΣ ΜΑΡΤΙΟΣ 2018
Georgios Bartzis
Department of Plant Sciences
Group of Mathematical and Statistical Methods – Biometrics
Wageningen University and Research Center
Estimating metabolite networks using a multi-step network approach that integrates information from lower leveled omic graphical structures
ΠΑΡΑΣΚΕΥΗ 30/3/2018
15:15
ΑΙΘΟΥΣΑ Τ105, 1ος ΟΡΟΦΟΣ,
ΝΕΟ ΚΤΙΡΙΟ ΟΠΑ (ΤΡΟΙΑΣ 2)
ΠΕΡΙΛΗΨΗ
In systems biology, an organism is viewed as a network of interacting molecular entities (genes, metabolites, etc.), and graphical structures are used for representing them. SNPs, genes, metabolites, or traits are depicted as nodes in a graph, and their associations by edges connecting them. Current methods recovering the graphical structure of multiple omic sources usually treat them independently and are limited in not using information from the structure of one to recover the other.
We develop a multi-step approach for reconstructing metabolite networks that borrows information from lower leveled omic sources (DNA or Gene level) that might have a graphical structure of their own. This multi-omic approach works from the lower (X) to the higher level (Y), e.g. from SNPs or Genes to metabolites in 3 main steps: i) recovers the graphical structure of X, ii) for every variable in Y utilises a regularised linear model using a network-constrained penalty accounting for the known data structure of X; the total variation of Y is separated into parts related to X and noise, and iii) reconstructs networks of Y using the part related to X variation. The main advantage is that the two omic sources are not treated independently and the graphical structure of the lower levelled source is explicitly modelled. This provides an additional level of information for guiding network estimation of Y on top of the integrated analysis.
We demonstrate our multi-step network approach in an Arabidopsis variant population (Bay-0 x Sha RIL), containing genetic variation related to gene expression, primary and secondary metabolite levels. In a first application, we work from DNA to metabolic level to find plant metabolites with similar genetic basis. In a second application, we move from DNA to gene level for estimating gene networks; then use the gene structure as input to estimate metabolite networks. Our results show that in many cases, metabolites are recovered more accurately due to using an extra level of information coming from the graphical structure of X (gene or DNA level).
- AUEB Stats Seminars 15/10/2021: Statistical Network Analysis with Bergm by Lampros Bouranis (AUEB)
- AUEB STATS SEMINARS 12/12/2018: A new approach to Bayesian image analysis by John Kornak
- AUEB STATS SEMINARS 10/5/2018: Interlocking directorates in Irish companies using a latent space model for bipartite networks by Prof. Nial Friel
- AUEB STATS SEMINARS 21/3/2018: Learning Markov Equivalence Classes of Directed Acyclic Graphs: an Objective Bayes Approach by Prof. Guido Consonni
- AUEB SEMINARS - 30/3/2016: Information fusion approach using signal quality indices, and information-theoretic feature selection: practical approaches and applications
Permissions in this forum:
You cannot reply to topics in this forum